
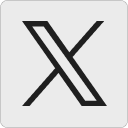



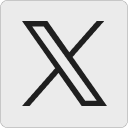


Poker isn’t like other games artificial intelligence has mastered, such as chess and go. In poker, each player has different information from the others and, thus, a different perspective on the game. This means poker more closely mirrors the kinds of decisions we make in real life but also presents a huge challenge for AI. Now, an AI system called DeepStack has succeeded in untangling this imperfect information, refining its own strategy to win against professional players at a rate nearly 10 times that of a human poker pro. We speak with Michael Bowling, who leads the team that designed DeepStack, to learn how.
RELATED: Artificial Intelligence Creates Magic Tricks
ResearchGate: What motivated this study?
Michael Bowling: Poker has been a challenging problem for artificial intelligence for decades. Chess and go have gotten more attention over the years, mostly because poker seemed beyond our reach. Chess, checkers, and go are games of perfect information, where both players have the same symmetric view of the game.
Poker is a game of imperfect information, where the players have different perspectives and knowledge about the play of the game because they can only see their own cards. This makes poker far more challenging. It also makes any resulting advances more applicable to real-life problems. It’s a rare moment indeed when we are faced with a decision where we feel we have all the information we need to make the correct choice. It’s far more common that somebody else holds information we need for our decision, or we hold information that someone else covets. AI advances in poker help us move AI toward tackling such problems.
RELATED: Learn about how chemists are using AI to speed up data work.
RG: What were the results of your study?
Bowling: We recruited 33 professional poker players and asked each to complete 3,000 hands of heads-up, no-limit Texas hold ’em against DeepStack. Our overall win rate, or how much money we are winning on each hand on average, is around 49 BB/100 (big blinds per 100 hands). This is an astonishingly high win rate. If the pros just folded each hand, DeepStack would’ve won only a bit more, at 75 BB/100. A pro player wants to maintain a win rate over 5 BB/100, and DeepStack was winning at almost 10 times that rate and against pro players themselves.
Furthermore, we can look at how individual players fared. Of the 11 players that completed the 3,000 hands, we beat all 11 of them. For all but one of them, the margin was statistically significant, meaning it is highly unlikely that we were beating them by just the luck of the cards.
RG: How did DeepStack achieve this?
Bowling: DeepStack makes a couple of fundamental advances. First, it avoids doing any abstraction—the process of grouping together different decisions in the game and pretending that they’re the same. This is traditionally how AI has dealt with large games of imperfect information. The problem with abstraction is that when you take actions, you are confused about what cards you are holding or how much money is in the pot or the size of bet the opponent just made. Any such confusion can leave a big hole in your strategy. DeepStack avoids abstraction by reasoning about each situation as it arises during play and computing its strategy for each exact situation.
The challenge of doing this reasoning in real time while playing is that there’s only a few seconds to figure out what to do. Reasoning from the current situation to the end of the game is all but impossible, unless it is very close to the end of the game. DeepStack doesn’t reason all the way to the end of the game, but rather reasons only a few actions deep—its action, the opponent’s response, its response back, and so on—before stopping and summarizing what will happen in the rest of the game using its “intuition.” This intuition needs to assign a value for how good it is to find yourself in different poker situations. By using its intuition, it never has to look very deep into the game to make a decision, making it possible to reason about what to do as if it were always close to the end of the game.
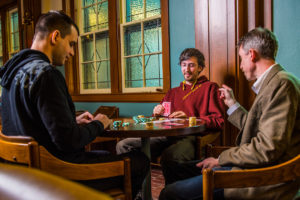
Finally, DeepStack’s intuition needs to be trained. Just like human intuition, it derives from the experience of other poker situations. Before playing against the pros, DeepStack saw millions of poker situations. In each one, it played against itself over and over again, refining its strategy, until it determined just how valuable it is to find itself in that poker situation. It then takes all of these millions of situations and trains a deep neural network, not to represent the value of these situations, but rather to be able to evaluate poker situations outside of this set. It generalizes its knowledge from its training situations to know ones it sees during play, just like human intuition.
Putting these pieces together, DeepStack reasons uniquely about each situation that arises during play. It reasons only a limited amount ahead into the game before using its trained intuition to evaluate how good it is to reach possible poker situations. This results in probabilities for each action it should take. When DeepStack must act again, it repeats this whole process.
RG: What type of computer do you need to run DeepStack?
Bowling: DeepStack can play at a high level without a whole supercomputer of computation behind it. In our study, DeepStack used only a single GPU to play, the kind of hardware you might find in a commodity gaming laptop.
RG: How will your study help advance AI more generally?
Bowling: Real-life decisions are much closer to poker decisions than to decisions in chess or go. Algorithms that can handle these types of situations make AI more generally applicable and open up many more areas for AI to have an impact.
One such area ripe for this sort of impact is in the allocation of security resources, for example, scheduling transit police to check for tickets in honor-system public transit or scheduling patrols to catch animal poachers. For these problems, you need to find schedules or policies that can’t be exploited by a malicious attacker, and they can often be formed as a sequential game with both sides lacking perfect information about the state of the world. We have taken a few small steps to apply techniques developed for poker to such settings.
Another less obvious application is for robust decision making, where one is concerned with the whole distribution of possible outcomes of one’s decisions rather than just the average outcome. This arises in financial risk management or even medical treatment recommendations.
Read more about the applications of AI: A New Metamaterial Sensor Classifies Spoken Words
A version of this article originally appeared on ResearchGate.
Featured image credit: David Singleton via Wikimedia Commons.

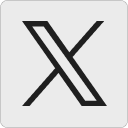



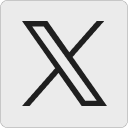

